The onion, the carrot, and the “alert budget”
.webp)
Get the Best of Data Leadership
Stay Informed
Get Data Insights Delivered
Did you know you already have a data quality budget? It might sound surprising, but you do.
I don’t mean a financial budget . I mean the total amount of time your team will spend in 2023 either fixing data issues, or hardening your data pipelines against various potential failure modes.
Every data team will spend some amount of time on the former (at least until the Metaverse delivers us from the realities of data engineering), and hopefully many will also allocate time to the latter. You can think of this budget in other ways - attention span, or capacity, or focus time.
So when you introduce a data observability product to your stack, be thoughtful about how you choose to roll it out. The rollout will impact how your team’s attention is directed, and therefore how your budget is allocated.
Two alert budget rollout options
Let’s say you have 1000 tables in your warehouse. We'll call the first rollout style the "onion" approach. It looks something like this:
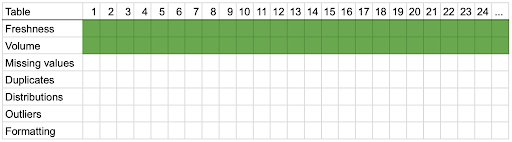
With this “onion” approach — your strategy is to monitor all 1000 tables for the simplest attributes like freshness and volume. Imagine peeling off just one or two layers from an onion. You get broad 360-degree visibility across your warehouse.
What happens to your team’s attention with this approach? They might be alerted to look at any table, anywhere, on any given day, to resolve a freshness or volume issue. Your organization should only roll out data monitoring this way if they use data very evenly, with only a few “hotspots” of regularly-referenced data. You trade breadth for depth.
Let’s call the second option the “carrot” approach. It looks something like this:
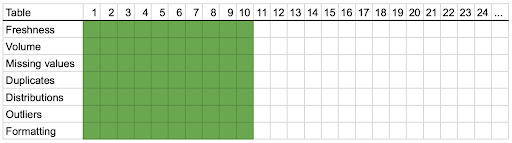
In this approach, you apply more fine-grained monitoring to a smaller number of tables. As opposed to the onion approach, where you’re lightly monitoring everything, you prioritize the highest-use tables in your organization. You leave the rest alone.
Imagine a tall and narrow carrot, wherein all tables are stacked on top of each other. The top 200 tables, the top of the carrot are highly to moderately important. The last 800 are infrequently used or unimportant. You monitor those top 200 tables for the same attributes, plus outliers, duplicates, and formatting problems. Your team will have more time available to spend improving the reliability of these 200 tables, at the expense of missing freshness and volume issues on the other 800.
Which approach do you choose?
Why do you need to make this tradeoff at all? Shouldn't you just monitor everything as deeply as you can?
No - you'd run out of your alert budget very quickly! Your time and attention are limited. Your data observability system needs to focus on the issues that impact data stakeholders (either internal ones like execs, or external ones like customers).
You have to be wise about spending your “alert budget.” You can loosely conceptualize the budget you have by following this formula:
(# Tables) x (# Attributes per table) = (Total Attributes)
If your observability system tracks seven attributes:
- Freshness
- Volume
- Missing values
- Duplicates
- Distributions
- Outliers
- Formatting problems
That gives you 7000 attributes that might draw your attention at any time (1000 tables x 7 attributes).
Your rollout strategy — onion or carrot — will determine which of the 7000 attributes you care about tracking.
There’s also another important factor to consider. There’s a non-zero false positive rate in any alerting system, whether it’s an ML anomaly detector error, or an erroneous set of manual threshold rules.
With a 2% false positive rate, in the broad-rollout scenario (the “onion”), expect around 40 useless alerts each day (1000 tables x 2 attributes x 0.02 = 40) spread across 1000 tables. With a 2% false positive rate in the narrow-rollout scenario (the “carrot”), expect 28 useless alerts per day (200 tables x 7 attributes x 0.02 = 28) across your top 200 tables.
Attention is precious, and your team can only respond to limited alerts each day. So take care with your “alert budget.” When it comes to data observability tools, you can choose between a broad and shallow “onion” approach, or narrow and deep “carrot” approach. There’s no right answer, but either way, make sure you’re safeguarding your team’s attention and focus.
Monitoring
Schema change detection
Lineage monitoring